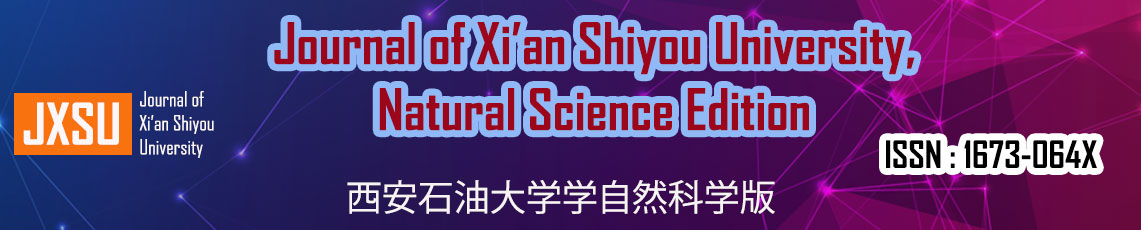
Home / Articles
Unveiling the Transformative Potential of Dark Data: An Optimized Extraction Method for Accurate Accident Prediction in Big Data
Amidst the era of data-driven decision-making, the persistent challenge of dark data—a reservoir of untapped information within routine transactions—necessitates the development of robust approach for its extraction. The exponential growth of big and dark data, particularly the escalating complexity of the latter, poses formidable challenges. This research introduces an optimized extraction method for accessing dark data using AdaBoost and Random Forest classifiers, showcasing transformative potential for precise predictions and uncovering latent insights. This method encompasses phases of analysis, implementation, and evaluation, aiming to address challenges in extracting insights from dark data. The evaluation of AdaBoost and Random Forest classifiers reveals AdaBoost's commendable overall accuracy of 78.4%, while the Random Forest classifier excels further with an impressive overall accuracy of 89.48%. This research pioneers the application of dark data in accident prediction, resulting in a substantial accuracy boost from 78.4% to 89.48%. Emphasizing the transformative potential of using dark data for precise predictions, the study underscores tangible benefits for decision-makers and urban planners. As data volumes escalate, the increasingly evident pivotal role of dark data in advancing decision-making and enhancing predictive model accuracy establishes a precedent for unlocking latent insights within previously untapped datasets.
Index Terms- Big data, Data quality, Dark data, Complexity of dark data, Accident prediction