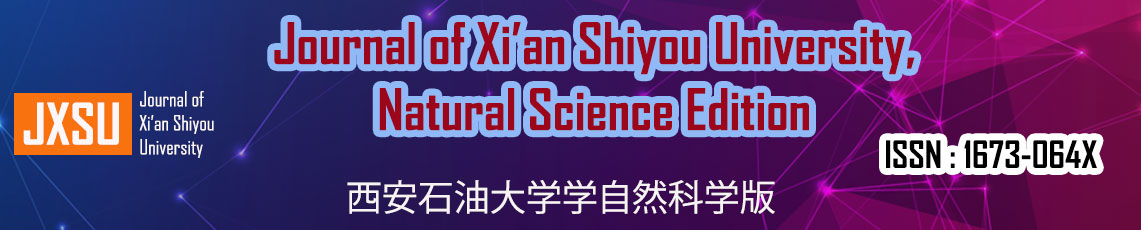
Home / Articles
iEnhancer-DNN: An Accurate identification of enhancer sites by heterogeneous feature using Deep Neural Network
Enhancers are the critical regulatory elements in DNA sequences that play an essential role in gene transcription and translation with in a genome. However, identifying enhancers is more complex than coding genes due to their high free scattering and positional variability. To address this challenge, numerous computational studies have been conducted in this field. Despite this, some deficiencies still exist in these prediction models. In this study, we propose a reliable computational approach for efficiently identifying enhancers based on a deep neural network model by incorporating heterogeneous features. The proposed model's effectiveness was evaluated using training and independent datasets through a 5-fold cross-validation approach. The validation results demonstrated that the iEnhancer-DNN model achieved an accuracy of 81.83%, respectively, when utilizing the training dataset. Similarly, when using the independent dataset, the model achieved an accuracy of 80.99%, respectively. Notably, our model outperforms previous methods in performance index, and providing valuable inspiration for the future of enhancer prediction using computer technology.
Keywords: Enhancer, DNA sequence, Identification, heterogeneous features, Deep neural network, Pseudo Dinucleotide Composition, Receiver Operating Characteristic, Matthews correlation coefficient