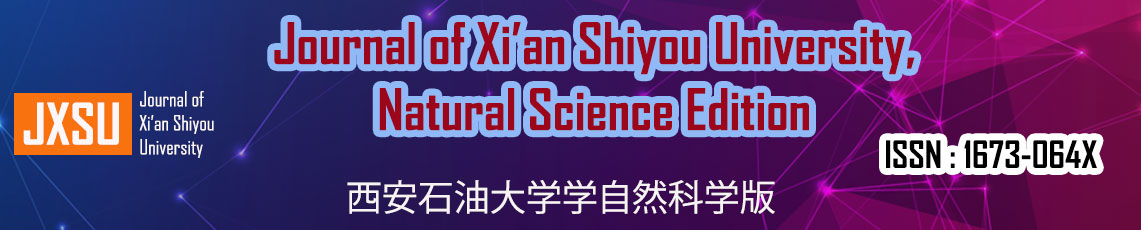
Home / Articles
iRhm5BiRNN: Identification of RNA 5-Hydroxymethylcytosine Modifications Using Bidirectional-Recurrent Neural Network
One of the essential areas of study in RNA epigenetics is the role of RNA 5-hydroxymethylcytosine (5hmC), which has been implicated in numerous biological processes. The identification of 5hmC can be achieved using multiple sequencing-based technologies; however, these existing approaches are time-consuming, expensive, and labor-intensive. Therefore, there is a significant need to develop more reliable, efficient, and feasible computational methods to replace or, ideally, complement high-throughput technologies. Despite developing different deep learning and machine learning models, their performance is currently insufficient and limited. In this study, we proposed a new identification scheme for deep learning, specifically a bidirectional recurrent neural network (BiRNN), called iRhm5BiRNN which overcomes the restriction of using only input information up to a present future frame for training. The BiRNN is trained simultaneously in both forward and backward directions, enabling it to accurately identify RNA 5hmC sites in genome-wide DNA sequences efficiently and reliably. Our approach utilizes a Bidirectional Recurrent Neural Network (BiRNN) to derive the most dependable features from the constantly changing RNA sequences. We achieved an accuracy of 85.51% on the benchmark dataset using our proposed architecture, surpassing the performance of existing methods in all evaluation metrics. These findings demonstrate the superiority of our approach and its potential to advance the field of RNA epigenetics.
Keywords: Deep learning, RNA 5-hydroxymethylcytosine, bidirectional recurrent neural networks, one-hot encoding