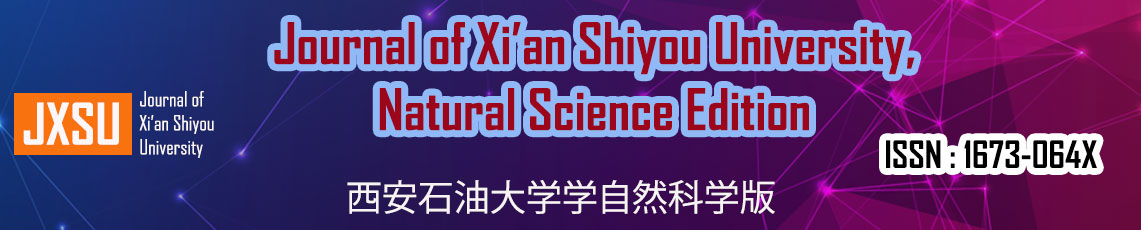
Home / Articles
Densenet169-based Deep Transfer Learning Technique for Rail Surface Defect Detection
Railway transportation plays an essential role in modern transportation infrastructure that requires the utmost safety and reliability. One of the significant concerns in railway management is the precise detection and timely repair of track surface defects, as these anomalies can lead to derailments, incidents, and disruptions in railway operations. Traditional inspection methods often rely on human expertise, which is susceptible to errors and time-consuming. In recent years, a growing interest has emerged in employing deep learning (DL) methods to automate the recognition and classification of faults in railway tracks. This paper aims to enhance the existing knowledge of this field by utilizing a convolutional neural network (CNN) based transfer learning model, DenseNet169, which can classify railway track images into defective and non-defective classes. In defective class, this research focuses on four defects, particularly: corrugation, flaking, shelling, and squats. In addition, data preprocessing and data augmentation techniques are also applied to overcome the challenge of class imbalance. The DenseNet169 results yield in high levels of recall, precision, F1-score, reaching cumulative accuracy of 97%. The developed system can serve as a complementary confirmation mechanism to reduce the likelihood of errors and improve the accuracy of surface fault detection in railway tracks.
Index Terms- Automated Machine Learning, Deep Neural Networks, Deep transfer learning, Densenet169, Rail Surface Defects.