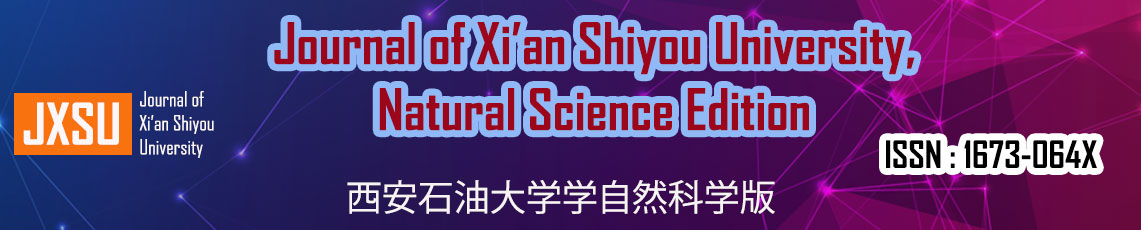
Home / Articles
DIABETES MELLITUS PREDICTION ON CLASS BALANCED DATASET USING XGBOOST ALGORITHM
With the advancement in the Information Technology and by the use of various Machine Learning techniques several models were built for predicting DM but majority of the algorithms exhibited an accuracy rate of 70%-90%. This clearly proclaims that still there is a need to build an efficient model capable of classifying distinctly. This paper aims at classifying the subjects into Diabetic and Non-Diabetic classes using the dataset drawn from the National Institute of Diabetic and Digestive and Kidney disease. SMOTE, an oversampling technique which overcomes the class imbalance problem is experimented on the dataset such that the classification dataset does not have a skewed proportion. The class balanced dataset is trained using the XGBoost algorithm, an ensemble technique akin to decision tree that makes use of Gradient Boosting framework out-turn an accuracy score of 97%.
Keywords – Diabetes Mellitus, XGBoost, SMOTE (Synthetic Minority Oversampling Technique)