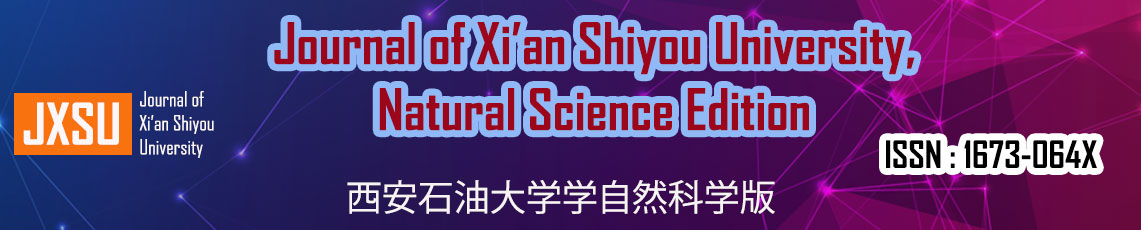
Home / Articles
Gesture Generation from Urdu Text based on Deep Learning Approach
When we interact with any machine-like robot or any other machine, gestures are important alongside speech. For years, efforts have been made to give computers human-like capabilities through research, but most gesture generation studies have been constrained by dependencies on speech input, the English Language, and specific speakers. This research aims to address these constraints through creating a gesture-generation model that generates high-quality gestures in return of Urdu textual input without requiring speaker assistance. We have developed our gesture model using Convolutional Neural Network (CNN) and Long Short-Term Memory (LSTM) algorithms, training it on a custom-created dataset. The implementation results demonstrate the model's effectiveness, achieving a Percentage of Corrected Key points (PCK) value of 75% and a mean absolute error rate of 0.3. These results validate that the model is successful in producing reliable gestures for AI interactions in the Urdu language. This research not only tackles the technical challenges of gesture generation but also advances the larger objective of enhancing AI's ability to interpret and respond to human nonverbal cues across different languages and cultural contexts.
Keywords:
Gesture Recognition; Natural Language Processing (NLP); Deep Learning; Urdu Text Analysis; CNN-LSTM Architecture.