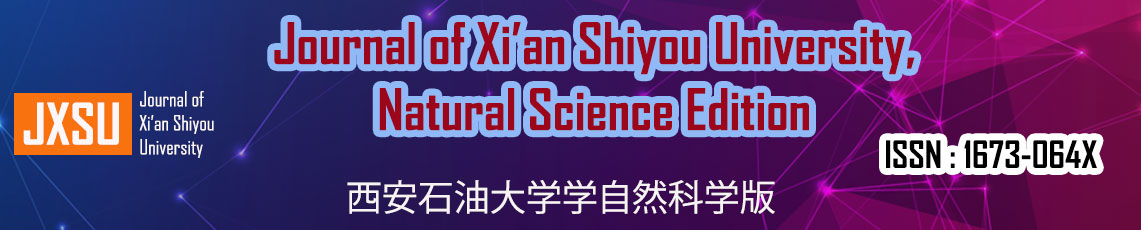
Home / Articles
Utilizing Retinal and Yawning Signals for Drowsiness Detection: A Comprehensive Approach through Machine Learning and Deep Learning
Globally, driving intoxicated is a significant factor as a major cause of accidents and presents a substantial hazard to public safety. In this research study, it is being suggested that a novel drowsiness detection system to tackle the growing problem, utilizing deep learning and machine learning methodologies. The proposed technique has multiple results, such as retinal and yawning inputs, which will enhance the detection accuracy and dependability of the system. The primary focus of this research is to utilize retinal pictures captured by cameras and analyze yawning episodes as physiological indications of fatigue or lethargy. A large dataset consisting of diverse samples from various circumstances is utilized to train and subsequently evaluate the model. The machine learning component utilizes traditional classification algorithms and feature extraction approaches to detect and analyze patterns in retinal pictures that are indicative of exhaustion and yawning. The architecture also has a deep learning component to reveal subtle details and intricate patterns within the data. Enhanced detection performance is attained by the automated utilization of Convolutional Neural Networks (CNNs) for extracting distinctive features from retinal images. In addition, recurrent neural networks (RNNs) are used to represent the temporal correlations of yawning sequences. This improves the system's ability to detect even the slightest signs of exhaustion. The efficiency of the suggested technology is evaluated through rigorous testing and validation in real-world circumstances. The system's ability to detect drowsiness is assessed by examining performance indicators, such as specificity, sensitivity, and accuracy. Furthermore, the proposed solution exhibits significantly higher resilience and detection accuracy compared to current methodologies. The findings of this study offer a comprehensive approach to significantly improve sleepiness detection systems by integrating analysis of yawning, retinal imaging, and advanced machine and deep learning techniques. If the proposed methodology is successfully integrated with vehicle safety systems, all parties involved would benefit from a reduction in the hazards associated with driver fatigue and an enhancement in road safety.
Index Terms- Drowsiness Detection, Machine Learning, Retinal Signals