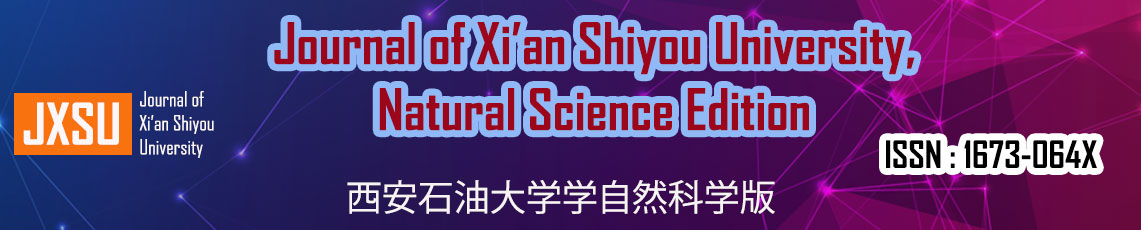
Home / Articles
Reinforcement Learning for Microgrid Energy Management
The consumer functions as an agent in a control strategy for solar microgrid energy management that employs optimization and learning to take optimal actions to minimize grid power usage through continuous interaction with the environment. Consumer behavior involves learning directly, allowing them to make decisions and take actions that are best for themselves in terms of scheduling. Consumers evolve through their interactions with environmental factors. This study examines a solar microgrid system that is connected to the grid and comprises a battery, a renewable generator, such as a solar photovoltaic system, and an energy consumer. A deep Q-learning-based energy management system, which is a Reinforcement Learning model, is used to maximize battery scheduling when solar power availability and dynamic load are involved. An algorithm for reinforcement learning is fed by the solar power and load. The solar microgrid operates optimally when the battery and solar power generator are both more useful and the electricity billing costs are reduced. The results of a system-dependability test using numerical data on price, cost, and load are displayed through simulations. The proposed energy management system accounts for the reduction in electricity bills from the main grid and uncertainty in the load and solar power.
Index Terms- Energy Management System (EMS), Microgrid, Reinforcement Learning (RL), Deep Q-Learning, Deep Q Network (DQN)