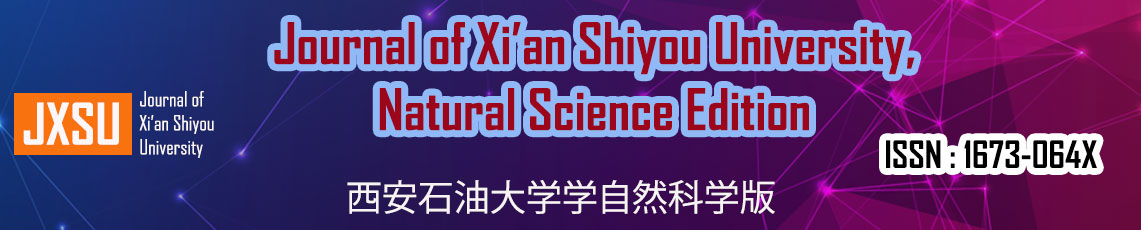
Home / Articles
Integrating EEG Signals and Machine Learning for Effective Driver Drowsiness Detection
Road safety requires driver drowsiness detection to prevent fatigue-related accidents. This study integrates EEG signals with facial landmarks to detect tiredness. The collection includes EEG data from drivers using Neuro-Sky mind wave headsets and image-processed facial landmarks. Preprocessing EEG signals and facial photos, extracting pertinent features, and then classifying with machine learning and deep learning models is the method. The dataset is analyzed using CNNs, LSTMs, XG-Boost, and Random Forest. EEG signal temporal dynamics and hybrid models to increase accuracy by mixing spatial and temporal information are studied.
Models like XG-Boost (79.99%) and LSTM (71.62%) show promising accuracy. Using multimodal data, the hybrid model with EEG and face landmarks outperforms solo systems. The study needs a larger dataset with more driving conditions and individual variances. The next steps include dataset enlargement, temporal dynamics investigation, hybrid model refining, and real-time implementation. For improved interpretability and proactive action, explainability and continuous monitoring should be integrated. This study shows how EEG signals and facial landmarks can detect driver tiredness. The findings emphasize the importance of multimodal techniques in enhancing accuracy and the need for continued research to solve problems and develop models for real-world applications. This work advances the development of robust and efficient sleepiness detection systems, improving road safety.
Index Terms- Driver drowsiness, Automobile accidents, sleep detection systems, Road safety.