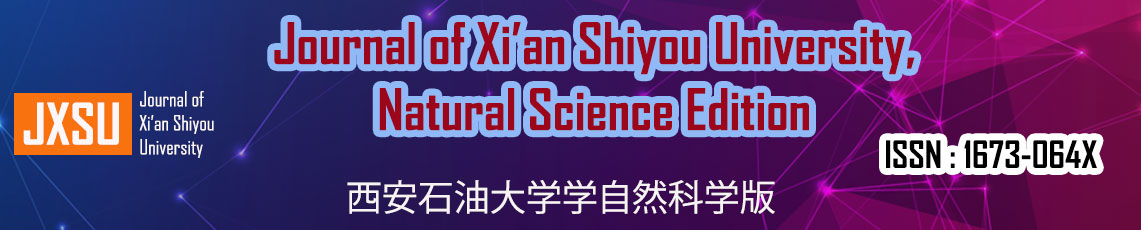
Home / Articles
Computational Methods for Detecting Transformer-Based Models Algorithm Fairness in Societal Bias Over Training Epochs
Social media sites play a significant role in shaping public opinion in today’s dynamic world. One of the challenges to these beneficial features of internet conversations is hateful sentiment. This research addresses the critical need to detect and mitigate hate speech, emphasizing the importance of fostering an inclusive and respectful online environment leveraging a Twitter dataset to explore hate-speech detection, classifying text into various categories of hate, violence, dehumanization, and demonization. Natural Language Processing (NLP) methods detecting hateful sentiment are tailored with unique challenges faced by people. The evaluation process involves rigorous testing of various models to assess their effectiveness in identifying hateful sentiment within social media sites such as X (Twitter) dataset. The proposed approach aims to contribute to ongoing efforts in combating hate speech within the online community. Analyzing the intricacies of hateful sentiment is imperative for safeguarding people freedom and establishing a digital environment that ensures safety and inclusivity in public discussions. The testing accuracies for LSTM achieved at 87.27%, BERT demonstrated a precision of 91.38%, DistilBERT exhibited a testing accuracy of 91.47%, RoBERTa performed at 91.05%, Hybrid RNN showcased an accuracy of 90.71%, and XLNet emerged with the highest testing accuracy at 91.68%.
Index Terms- NLP, Hateful Sentiment, Detection, BERT, XLNet