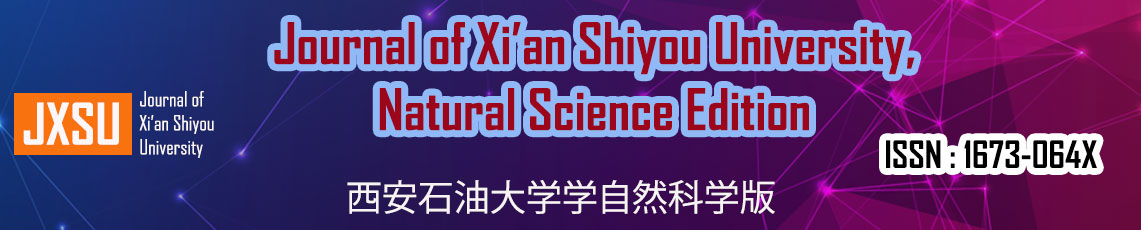
Home / Articles
Deep Hybrid Parallel CNN-LSTM Model for Diabetes Prediction Using Fusion of Features
Diabetes is a persistent disease that has high prevalence and causes various complications. Using EHR to identify the individuals with the high risk of developing diabetes could improve quality of health care. This paper represents a hybrid deep model and unique engineered features to forecast diabetes. Hybrid deep model merge Long-Short Term Memory (LSTM) with Convolutional Neural Networks (CNN) and also utilizes the relative statistical data. Unlike the traditional stacked CNN-LSTM, CNN and LSTM in the proposed model extract the features in parallel and are more informative. CNN uses three filters to extract temporal features at different level using convolution technology, whereas LSTM extract the sequential relation using past visit records. These extracted features are merged with corresponding statistical data. Overall the resultant feature is the combination of multi-type (statistic and time domain) and multi-level. Moreover, the model performance is tested for two training density. Result represents the state of art performance of the proposed model for both the training samples.
Keywords- Diabetes, EHR, Hybrid Deep Model, Long-Short Term Memory (LSTM), Convolutional Neural Networks (CNN), stacked CNN-LSTM, multi-type, multi-level.