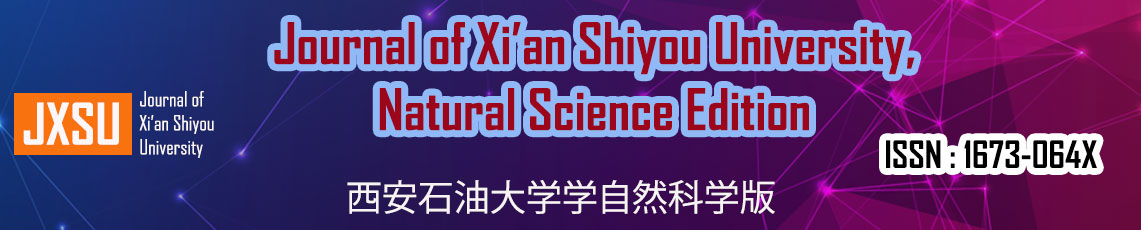
Home / Articles
Enhanced Glaucoma Diagnosis Through U-Net Deep Learning and DenseNet-201 Feature Extraction
Glaucoma exhibits a significant prevalence across multiple nations, with a notable increase in prominence observed within the United States and Europe. According to recent data, the global prevalence of glaucoma stands at approximately 78 million individuals as of the year 2020. Projections indicate that this figure is expected to rise significantly to reach an estimated 111.8 million by the year 2040. In regions characterized by inadequate healthcare infrastructure aimed at addressing glaucoma, it has been observed that the condition is misdiagnosed in a staggering 90% of cases. In order to optimize the identification of early-stage glaucoma, it is crucial to develop a reliable and effective detection system. In the present study, we propose the adoption of deep learning technology as a means to detect and forecast the onset of glaucoma prior to the appearance of symptomatic indications. The utilization of a deep learning algorithm is predicated upon the utilization of a glaucoma dataset for the purpose of conducting image analysis. In order to enhance the efficacy of optic cup segmentation through the utilization of deep learning principles, the integration of pretrained transfer learning models with the U-Net architecture is seamlessly executed. In the context of feature extraction, the DenseNet-201 deep convolutional neural network (DCNN) is utilized. The utilization of the DCNN methodology plays a pivotal role in the identification and assessment of glaucoma in individuals. The principal aim of our research endeavor is to discern the presence of glaucoma in retinal fundus images, thereby enabling the evaluation of a patient's ocular health status. The bidirectional impact of glaucoma on the model results in outcomes that could vary in either a positive or negative direction. Metrics such as accuracy, recall, precision, F-measure and specificity, the F-score are of utmost importance when assessing the performance of a model. Furthermore, a comprehensive comparative analysis is undertaken in order to determine the level of accuracy exhibited by the proposed model. The results are contrasted with classification techniques utilizing convolutional neural networks that are rooted in deep learning principles. During the training phase, our developed model demonstrates a commendable accuracy rate of 98.82%. Subsequently, during the testing phase, the model exhibits a notable accuracy rate of 96.90%. Extensive evaluations consistently validate the superiority of the proposed paradigm over the presently employed methodology.
Index Terms- Glaucoma, Image Segmentation, U-net, Dense Net 201 Model.