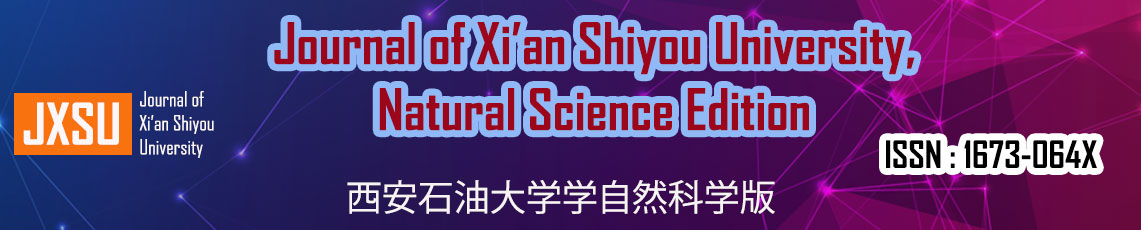
Home / Articles
Detecting Malicious Emails: Machine Learning Techniques Unleashed
This research investigates novel methods for effectively detecting malicious emails using machine learning techniques. Recognizing the evolving landscape of cyber threats, the study delves into advanced methodologies, employing sophisticated algorithms to discern subtle patterns indicative of malicious intent within email content. The research emphasizes a unique combination of feature engineering and leveraging diverse attributes. Through a comprehensive analysis of a diverse dataset, the study showcases the superior efficacy of the proposed machine learning model in accurately identifying and mitigating the risks associated with malicious emails. The findings contribute to the ongoing discourse on cybersecurity, presenting novel insights and advancements in safeguarding digital communication channels against evolving cyber threats.
Our research endeavors involve the exploration of an effective model for identifying malicious emails, utilizing eight diverse datasets across various dimensions. We employ different feature engineering techniques, including term frequency-inverse document frequency (TF-IDF), count vectorization (CV), and a combination of both TF-IDF and EPOCH. Additionally, we integrate the top three models through stacking and rigorously evaluate the outcomes to enhance our understanding of mitigating phishing threats effectively.
Index Terms- Cybersecurity, Data Analysis, Detection, Classification, Machine Learning, Malicious Emails.