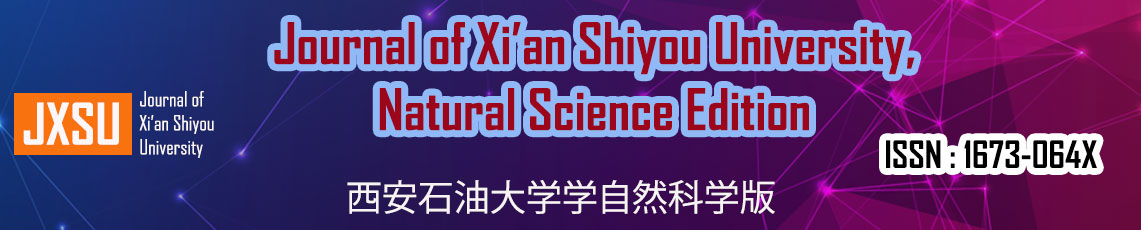
Home / Articles
Perceived Stress Detection through EEG Data Segmentation and Classification
Human stress is an increasingly prevalent and pervasive issue in modern society, posing significant challenges to individual well-being and overall societal health. There are many stressors in daily life that can trigger serious health issues, current study is focused on perceived stress. This paper aimed to investigate the correlation between electroencephalography (EEG) and Perceived Stress Scale (PSS) by utilizing data segmentation technique. The procedure involves: data acquisition, preprocessing, data segmentation, feature extraction and selection, and classification. The PSS scores were employed to record perceived stress levels of individuals. These PSS scores served as the basis for categorizing the data into two: stressed and non-stressed, and alternatively, into three classes: stressed, mildly stressed, and non-stressed. EEG recordings were captured from 40 participants (healthy and free from any known mental disorders) using 4 channel Interaxon Muse headband that was equipped with dry electrodes. The EEG data was then segmented into units of 10 seconds. The data was processed to extract five feature sets. These sets include Power Spectrum (PS), Rational Asymmetry (RASM), Differential Asymmetry (DASM), Correlation (CR) and Power Spectral Density (PSD). The stress level was accessed utilizing four classifiers: Multi-Layer Perceptron (MLP), AdaBoost M1, Random Forest and Bagging. The results indicate that AdaBoost M1 and Random Forest classifiers predicted the two classes with maximum accuracy levels of 91.52% and 88.47% for two- and three-class stress classification, respectively. These findings underline the importance of the chosen features and classifiers in increasing the prediction accuracy while contributing to the existing knowledge on stress detection with EEG signals.
Index Terms- Feature Extraction, EEG signals, Perceived Stress, Stress Detection