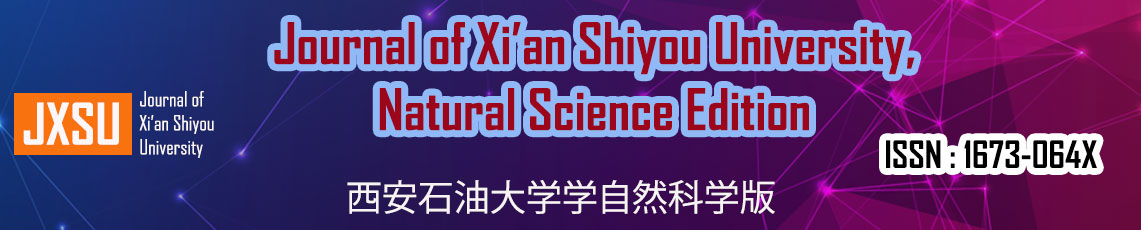
Home / Articles
A Comprehensive Review of Sensor-based Sign Language Recognition Models
Sign language is a visual language used by millions of people worldwide who are deaf or hard of hearing. Sign language recognition (SLR) has been an active research area in the field of human-computer interaction for several decades. Sign Language, which is a combination of hand and facial gestures, is used as a method of articulation by this community. However, not everyone knows these gesture languages. Thus, deafness and loss in hearing capabilities impacts their ability to communicate, which further leads to social isolation. SLR is essential to build an inclusive society. SLR helps to automate the conversion of SL to text or voice. With the availability of low-cost sensors and advancements in machine learning techniques, there has been a growing interest in using sensor data for SLR. Sensor-based SLR (SSLR) has the potential to improve the accuracy and robustness of the recognition system and enable the development of portable and wearable SLR devices. This review paper provides a comprehensive overview of the existing techniques for SLR using sensor data. The paper discusses the data acquisition, preprocessing, feature extraction, selection, and machine learning models used in SSLR. The review paper also highlights the challenges and limitations of the existing techniques and provides insights into future research directions and opportunities.
Keywords - Sign Language Recognition, Human Computer Interaction, Sensor-based, Feature Extraction, Machine Learning