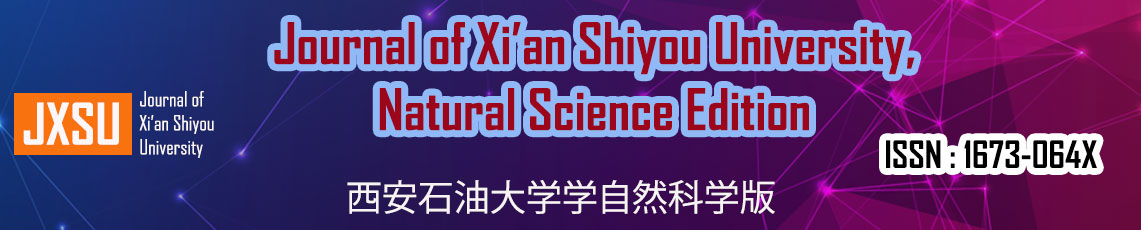
Home / Articles
KL Grade Classification of Knee Osteoarthritis using Convolutional Neural Networks and CBAM
Knee osteoarthritis is a prevalent musculoskeletal disorder that predominantly affects the elderly population and is typified by the gradual deterioration and attrition of articular cartilage in the knee joint. One commonly employed technique to evaluate the extent of knee osteoarthritis is the Kellgren-Lawrence (KL) grading system, which evaluates joint space narrowing, osteophytes, sclerosis, and bony deformity visible on radiographic images. The purpose of this study is to compare and contrast the Knee Osteoarthritis KL grade classification performance of various architectures of Convolutional neural networks including ResNet-34, DenseNet-121, VGG-19 and Inception-V3. The Osteoarthritis Initiative (OAI) dataset was chosen as the primary dataset for our research. In this study, osteoarthritis is classified solely based on radiographs (X-RAY) of the knee obtained in a bilateral posteroanterior projection with fixed joint flexion. We investigate the effectiveness of the Convolution Block Attention Module on these architectures for knee osteoarthritis severity level. We merged KL scale grades 0 and 1 into a single category which resulted in a 11.84 % improvement in accuracy without losing any essential data. We observed that DenseNet-121 with CBAM performed the best among all the models, achieving an accuracy of 86.714% with highest per class accuracy of 93.15% achieved on Grade 0.
Index Terms- Knee Osteoarthritis, Kellgren-Lawrence scale, Convolution Block Attention Module, DenseNet, ResNet, VGG, Inception