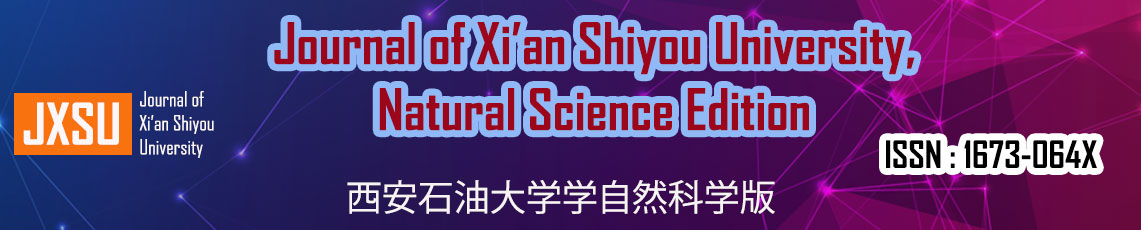
Home / Articles
STOCK PREDICTION USING FINANCIAL DATA AND NEWS SENTIMENTAL ANALYSIS
Predicting stock market prices has long been a subject of concern to investors as well as academics. Because of their extremely volatile existence, which depends on numerous political and economic influences, leadership transition, investor sentiment and several other influences, stock prices are hard to predict. Predicting stock prices based on either historical or textual data alone proved inadequate. Current studies in sentiment analysis have found a strong link between stock price movement and the publishing of news papers. Several studies of sentiment analysis were attempted at different stages, using algorithms such as supporting vector machines, naive regression of Bayes, and deep learning. The accuracy of deep learning algorithms depends on the amount of data given for the training. However, the amount of textual data obtained and analyzed during the past studies was inadequate and thus led to low accuracy predictions. In our paper, we are improving the accuracy of stock price forecasts by collecting and analyzing a large amount of time series data in relation to relevant news storey’s, using deep learning models. For five years, the dataset we have compiled contains daily stock prices for S&P500 firms, along with more than 265,000 pieces of financial news related to those firms. Because of the large size of the dataset, we use cloud computing as an indispensable resource to train prediction models and perform inferences in real time for a given stock. Index Market prediction terms-stock, cloud, big data, machine learning, regression.
Keywords:Companies, models, Data, Feature Extraction, Facebook, Recurrent Neural Networks, Stock Markets